Personalized healthcare and precision medicine are roping in cutting-edge technologies, but their path is strewn with technical, political, and regulatory challenges.
Be sure to check out Part II, Part III, Part IV, and Part V of our interview series on precision medicine!
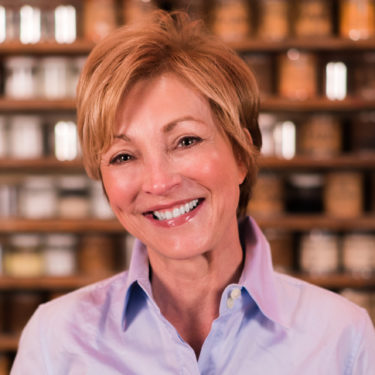
Kathy Gibson
“A number of technical, political, and regulatory challenges remain to leveraging precision medicine for deep learning applications on a large scale, which may hamper rapid progress”
Kathy Gibson, Chief Innovation Officer at CAS, tells The Sociable that application of big data technologies such as deep learning and AI to precision medicine is “really just in its infancy when it comes to clinical application.”
“In this age of electronic medical records, a large volume of data is being gathered. Still, a number of technical, political, and regulatory challenges remain to leveraging precision medicine for deep learning applications on a large scale, which may hamper rapid progress,” she says.
Customized medical approaches for individuals or small populations is no doubt resulting in volumes of analyzable data. AI and deep learning are successfully helping in this analysis, for example, interpreting diagnostic imaging scans.
However, to be successful, these models require large volumes of high quality, normalized data that fully represent the breadth of the population that the models being developed are intended to be applied.
Real-World Precision Medicine Application
One of the biggest hurdles to real-world application of these technologies is that electronic medical records today are stored in many different formats, structures, and languages, requiring a lot of work and collaboration to build a homogeneous data set.
Read More: Deep tech, big data, and their impact on precision medicine
Gibson says this challenge has been a focus for CAS partnerships with healthcare R&D organizations that leverage CAS’ content collection, scientific data management expertise, and technology to augment and normalize their data collections for use in AI and machine learning projects.
No Guarantees For Personalized Healthcare Yet
Testing for genetic mutations is becoming the standard of care for melanoma, lung cancer, and a few other tumor types. Those targeted therapies help about half of the patients that receive them.
Still, Gibson says the number of people with advanced cancer that are actually eligible for these tests is estimated to be less than 20%.
“While real progress has and continues to be made, we are certainly not at a point where a genomic test can be evaluated, and treatment identified with a guaranteed positive outcome,” she says.
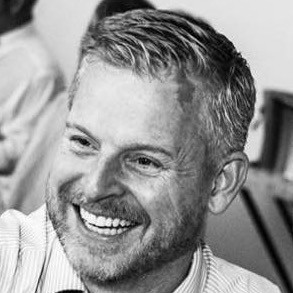
Matt Lashey
“Every patient is unique. Their genetic make-up, along with a plethora of other factors can have a major impact on how they respond to treatment”
Matt Lashey, Founder and CEO of health tech startup Treatment Technologies & Insights (TTI), told The Sociable that genetic information is a key part of the puzzle to understanding the efficacy and potential risks of treatment options for each patient.
However, there are also a lot of other patient activities, experiences, and lifestyle factors that can influences drug efficacy and risks as well.
“I think many of the treatments for chronic conditions are standardized. However, every patient is unique. Their genetic make-up, along with a plethora of other factors (environmental, lifestyle, mental, etc.) can have a major impact on how they respond to treatment,” he says.
TTI is also the creator of ChemoWave, a mobile application that makes it easy to record and monitor every aspect of a chemotherapy experience, and helps patients and caregivers understand how actions and experiences impact the ups and downs of a patient’s condition.
AI Bias in Precision Medicine Data Sets
AI bias is certainly a risk that must be actively managed. Gibson says the primary source of bias in AI applications would likely come from biases and limitations in the data sets that are used to build and train the underlying algorithms and models.
“It is critical that the training sets for these applications have high accuracy and fully represent the populations that the final model is going to be applied to, otherwise the conclusions may be inaccurate,” she says.
Read more: Machine learning will be able to predict diseases years before onset of symptoms
For example, if a model to prioritize treatment options for cancer patients was trained on a data set consisting of only information on adult males aged 40-60 years, and then the model was applied to personalize treatment for a 12-year old female child, there is a high likelihood that the recommendations would be inaccurate due to the differences in these populations.
Precision medicine is undoubtedly heralding in an innovative approach by taking into account each individual’s different characteristics.
However, as technologies like big data, AI, and deep learning partner to achieve precision medicine’s goals, some fine-tuning is still required in order to reach the masses.